Automation in the industrial sector is advancing inexorably. A high degree of networking and digitalization is required along the entire value chain. For this, investments in the right technologies are indispensable. Machine vision is an area that offers enormous potential. However, companies are often reluctant to make use of this potential. A lack of qualified personnel and a lack of programming skills among the employees are frequently cited as reasons for not using machine vision. But this does not have to be the case: Thanks to easy-to-use machine vision software, any company can easily and quickly leverage the benefits of machine vision – without any programming skills.
Most industrial sectors today rely on a very high degree of automation in their value chains. To meet the requirements of industry 4.0 and the smart factory, production processes must be networked and digitalized end-to-end. Machine vision can make a valuable contribution in numerous areas. Functioning as the “eye of production,” it monitors all manufacturing, quality assurance, and intralogistics processes. This involves two components: software and hardware, in the form of image acquisition devices such as cameras or sensors strategically placed in the production environment that continuously capture and generate substantial volumes of digital image data.
Easy-to-use
The machine vision software which is connected to the image acquisition devices processes the provided information almost in real-time and makes it available to superordinate quality management and plant control systems, enabling high levels of automation. However, the integration of professional machine vision applications is usually very complex. Here, easy-to-use machine vision software can provide a solution. Since no programming skills are required for such machine vision software, industrial image processing can thus also provide a valuable contribution to digitization for small and medium-sized companies in various industries, where qualified personnel or corresponding programming skills are often scarce.
Software can also address the increasing importance of artificial intelligence (AI) in the industrial context with its integrated deep learning technologies. Using self-learning algorithms, outstanding results can be achieved in object and defect detection. If neither the amount of high-quality image data, nor the powerful hardware required for training are available, or if one is dealing with very high production speeds, classical rule-based methods are often the better alternative.
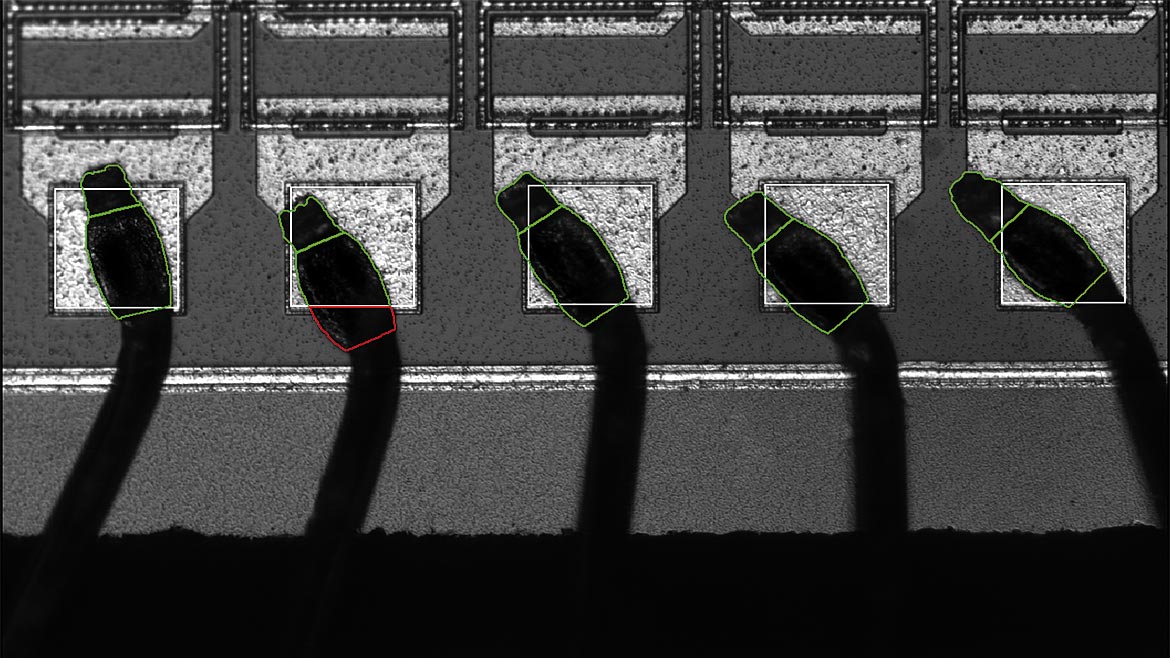
What can machine vision do?
The technology is indispensable for modern, highly automated inspection processes as part of operational quality assurance. It can be used to reliably detect any type of defect in objects. The faulty products can then be automatically classified as rejects and sorted out before they enter the subsequent process chain. In addition, objects can be measured precisely to ensure the correct dimensions and thus, the required product quality. Using optical character recognition (OCR) technologies, objects are recognized not only based on shape and texture, but also based on imprinted combinations of numbers and letters. Machine vision not only enables precise positioning and alignment of workpieces but also aids robots in gripping, handling, and depositing objects accurately, eliminating the need for direct operator intervention. This automation enhances the efficiency and safety of the entire handling process.
Whether in traditional manufacturing, automotive production, machinery and plant engineering, electronics manufacturing, battery production, or even the food and beverage industry – machine vision can be used across these and many other industries. It not only operates very quickly, but also very precisely. Processing large amounts of digital image data takes place in a few milliseconds, providing very accurate, reliable, and robust results. Thus, thanks to modern machine vision, companies can save significant costs – whether in assembly, quality assurance, or logistical workflows.
Software in practice
Two examples from different industries show the advantages of using machine vision in the industrial sector.
Vision & Sensors
A Quality Special Section
In the food industry, products such as potatoes or chocolate bars are usually unsorted on the conveyor belt when they are transported to the robot packing area. To enable the robot to precisely grip the products in this chaotic scene, software can detect the products with the help of a camera and transmit precise position data to the robot. Once the food items enter the robot’s work area, it starts handling the products based on the data from the software, following the first-in/first-out principle. The machine grabs the front most product and deposits it in the designated location. The coordinate systems of the software and the robot are aligned so that the vision coordinates precisely correspond to the robot’s trained workspace. To ensure that the robot can find the objects precisely, robustly, and quickly even under difficult conditions, software’s integrated matching technology is used. This allows the robot to flexibly respond to changing variables, such as when contours are rotated, scaled, perspectively distorted, partially covered or outside the image.
Especially when inspecting complex parts, such as metal springs, deep-learning-based technologies like global context anomaly detection yield excellent results. In this process, a powerful camera captures an image from above for each component. On the captured images with the metal springs, global context anomaly detection performs the inspection. The deep learning technology consists of two neural networks. The “local” network checks for small-scale defects such as scratches, cracks, or contaminations. The “global” network goes one step further and checks for logical defects, such as bent or missing brackets. From the interference of the two networks, global context anomaly detection determines an anomaly score. This score is then compared to a pre-defined anomaly threshold. If the anomaly score is above this threshold, it indicates a defective component that is rejected. The inspection process using deep learning technology provides a major advantage over using a rule-based method. In a rule-based approach, all possible types of defects must be individually extracted and defined based on “bad images.” In contrast, for training software’s deep learning methods, only “good images” are needed.
Machine Vision for everyone
Machine vision provides significant added value for companies of all sizes and industries. Thanks to easy-to-use software, the implementation and utilization are straightforward and quick, allowing for processes optimization and employee relief.