Since the economy climbed out of the last recession, “Help Wanted” signs have become a common fixture near manufacturing facilities all over the United States. With 10,000 baby boomers reaching 65 each day, retirements are leaving a significant experience gap to be filled.
In a recent report from the Automotive Industry Action Group (AIAG), concerns having the biggest impact on the quality universe over the next few years include a lack of problem-solving ability and a loss of experience. From our perspective, as experienced workers retire and walk out the door for the last time, the problem-solving skills and institutional knowledge will continue to decline.
While there are no easy answers to these concerns, finding ways to improve problem-solving skills would be a significant win for manufacturing. These skills can be leveraged in a number of areas, including increasing operational efficiencies, monitoring and responding to quality-related events in a timely manner, and improving brand and customer relationships.
I am guessing many of you have intelligent helpers – think Siri, Alexa, Cortana, and others – in your homes. They represent a portion of the much-talked-about “dawn of artificial intelligence.” One of my favorite science fiction authors noted that the future is already here, it just isn’t evenly distributed. It seems like quality often lags behind the rest of the world in technology implementation, so this statement isn’t surprising to me. In fact, one of my clients often speaks of her company’s (unwritten) policy of “Yesterday’s technology tomorrow” for the quality department!
So, how can those of us in the quality software and consulting business help with these issues? One common observation is that the lack of experience with a manufacturing process results in repeatedly solving the same problems. To counter this, an electronic system may be put into place that helps with triage and diagnosis. If a problem occurs on the plant floor, this “manufacturing emergency medic” can be quickly dispatched at the problem area. Armed with a simple series of yes/no questions that have been vetted by more experienced personnel, even a rookie can often quickly get to the root cause of the problem. Given reduced headcount in the quality department, retirements, turnover, and insufficient training, a software assistant like this can be a great help. Additionally, this type of system can provide a repository of historical data about troubleshooting steps that have resulted in a successful diagnosis. Maybe step 5 turns out to be a remnant of a past system, never actually solves the issue, and should be removed from the current troubleshooting process.
This codification of corporate knowledge should be a priority for today’s companies. Recording experienced employees’ tips and tricks in documents that can be delivered electronically on demand will result in less confusion on the part of new operators. Creating a database of response protocols and tracking how well they work will build a valuable corporate resource. Exit interviews should be utilized to help build up this knowledgebase, and an effective apprentice program should utilize this information as new employees get up to speed more quickly. When that invaluable, experienced employees walks out the door for the last time, much of their knowledge and problem-solving skills can stay behind and continue to benefit the company.
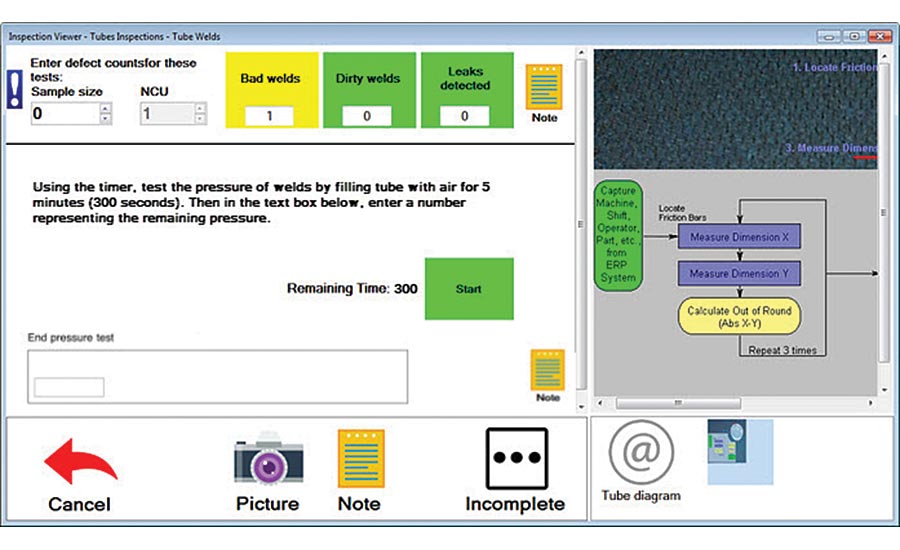
Screenshot of inspection view for tube welds. Source: Hertzler
Now imagine how those benefits can be multiplied by sharing those experiences, best practices and problem-solving gains across the supply chain. When all your plants use the same software, workflow, inputs, and knowledge in the same way – when the quality function is standardized across the corporation – all of your most experienced problem-solvers contribute to a shared base of knowledge for addressing quality issues. Moreover, because data is stored in a standardized format, managers can analyze that data to determine which products run most effectively on certain lines at certain plants. The results of drilling down into line and plant efficiencies for different products can be quite an eye opener. Sharing of best practices from the best-performing lines can thus become a critical component of corporate continuous improvement efforts.
Software wizards can also assist in identifying root causes. Whether looking at defect data or measurement data, collecting associated traceability information with the raw data opens up the possibility of the software identifying the sources of variation in a process. Whether it is interactive grouping, slicing and dicing of data by an engineer, or a wizard processing “big data” sets that runs in the background and suggests the top issues based on statistical calculations, there are plenty of opportunities to get assistance from our benevolent computer overlords!
One especially exciting project that I was involved with recently consisted of setting up a table that relates tools and grinding wheels to the product features that they impact. As the product dimensional values trend upward or downward due to tool wear or positioning issues, the software predicts how many more parts may be machined before the in-control system will start producing out-of-spec parts. This system goes beyond predictive statistics, though, and moves into the prescriptive realm by suggesting which tool should be dressed or changed next to each feature’s trend chart. Displaying the number of parts before the out-of-specification failures will begin occurring helps keep the operators in the loop. In some cases where cycle times are consistent, the software may display the amount of time before intervention will be needed, rather than a number of parts. Hints as to the action needed are especially helpful for new operators and they are grateful for the assistance.
Artificial intelligence can also help to close the loop on building the knowledge base of problems experienced on the shop floor – and their solutions. You can build this AI into your operators’ workflow to help them enter better notes when a problem occurs. There are two common approaches to improving note entry. The first method determines the probability of the note belonging to a pre-defined “good” set of notes. It then becomes a simple task to pop up a warning to the operator to review the note text if the probability falls below a certain value. The second method determines similarities to individual notes in the pre-defined set. Often, the two methods are combined in analyzing the note, e.g. the note has a greater than 90% chance of being in the “good” set based on the words used and is similar to (or matches) a pre-defined “good” note.
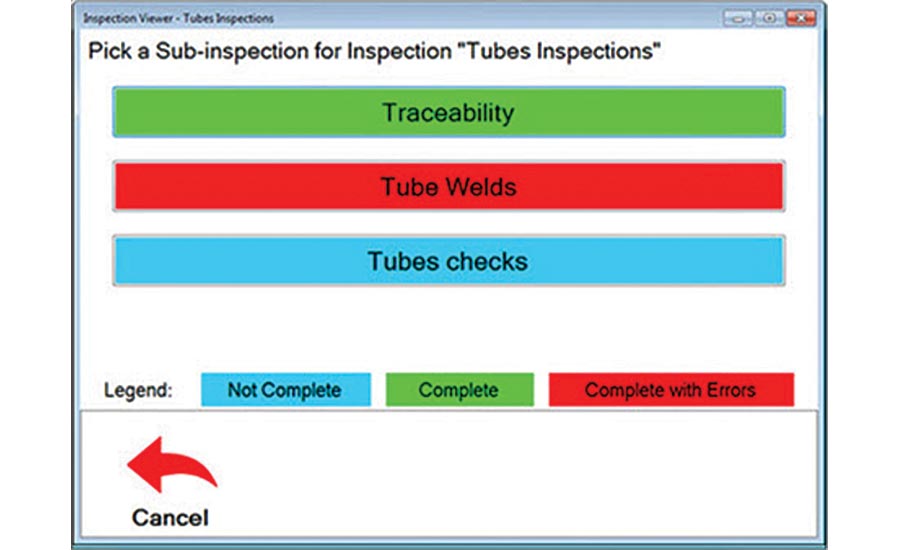
Sub-inspection screenshot for tube weld project. Source: Hertzler
These AI algorithms may also be applied to notes after the fact to determine if the notes achieve an acceptable standard. In some cases, an operator’s monthly bonus might be tied to entering meaningful notes. For example, Mary and Martha both enter 60 to 70 notes a month, but post-processing their notes through the AI algorithms determines that only about half of Mary’s notes are considered legitimate (too many “asdf” entries when she is in a hurry). On the other hand, Martha’s notes are consistently above a 95% acceptance rate, which is the minimum required to achieve the monthly bonus. Automating the task of auditing the notes reduces the amount of time a supervisor needs to spend reviewing the notes. It also encourages the operators to enter meaningful notes, thus contributing to the overall process knowledgebase.
It might not seem like HAL or Siri, but AI is surely and slowly making its way into our work lives. State-of-the-art tools can and should be utilized to maximize your quality efforts, even on the manufacturing floor! Q